What’s Under the Hood of an AI Agent?
If an AI agent is the brain, then its architecture is the blueprint. Just like buildings need a solid structure, AI agents need the right architecture to function effectively in their environments. From the no-nonsense reactive agents to the ambitious hybrid thinkers, each design comes with its own style, strengths, and strategy. Let’s take a guided tour through the key agent architectures in AI, how they differ, and when to use what.
Reactive Agent Architectures in AI: Fast, Focused, and Forgetful
Let’s start with the speed demons of the agent world.
🔧 How They Work
Reactive agents operate on condition-action rules. They don’t think too hard—they just react. No memory. No learning. No fuss.
- “If I see a wall, I turn.”
- “If there’s danger, I flee.”
🧠 Real-World Example
- Roomba: Your robotic vacuum that turns when it hits furniture? Classic reactive behavior.
- Simple bots in games: Think of Goombas in Mario—walk until something hits them.
✅ When to Use
- Environments are fully observable and simple.
- Speed is more critical than strategy.
⚠ Weakness
They lack adaptability. They don’t plan, remember, or improve over time.
Model-Based Reactive Agent Architectures in AI: Reflexes + Memory
Reactive agents with a touch of intelligence.
🔧 How They Work
These agents keep an internal model of the world. They still react quickly, but now they consider past perceptions too.
- “I saw this wall earlier. It’s still there.”
🧠 Real-World Example
- Smart thermostats: They adjust temperature based not only on current readings but also on previous behavior and patterns.
✅ When to Use
- Environments are partially observable.
- Agents need some memory but not complex reasoning.
⚠ Weakness
They still don’t plan for the future. They react slightly smarter, but that’s about it.
Goal-Based Architectures: The Planners
Now we’re getting strategic.
🔧 How They Work
Goal-based agents don’t just act—they plan. Given a goal, they search through possible actions to achieve it.
- “I want to reach Point B. What’s the best way from Point A?”
🧠 Real-World Example
- GPS Navigation Systems: They don’t drive for you, but they plot the best route to your destination.
- Chess AIs: They explore multiple moves to reach a win state.
✅ When to Use
- You need agents that make decisions based on defined goals.
- Planning time is acceptable.
⚠ Weakness
Planning takes time and resources. Not ideal for real-time reactions.
Utility-Based Agent Architectures in AI: Decision-Makers with Taste
Why just reach a goal when you can reach the best goal?
🔧 How They Work
Utility-based agents rank different outcomes using a utility function. They don’t just succeed—they optimize.
- “Both routes reach the goal, but this one avoids traffic and is safer.”
🧠 Real-World Example
- Netflix Recommendation Engine: It doesn’t just give you any movie—it predicts what will give you maximum satisfaction.
- Autonomous Cars: They consider fuel usage, time, safety, and comfort to choose actions.
✅ When to Use
- Multiple goals or trade-offs are involved.
- You want smarter, richer decision-making.
⚠ Weakness
Defining the utility function can be tough. Also, more computation = slower reactions.
Learning Architectures: The Evolvers
Meet the agents that learn, adapt, and get better over time.
🔧 How They Work
Learning agents consist of four components:
- Performance Element (does the task)
- Learning Element (improves the agent)
- Critic (gives feedback)
- Problem Generator (tries new things)
- They evolve through experience and feedback.
🧠 Real-World Example
- Self-Driving Cars: Improve with every mile driven.
- AI Chatbots: Learn better responses through user interactions.
✅ When to Use
- Environments change over time.
- Long-term performance matters.
- Data is abundant.
⚠ Weakness
Learning can be slow, and early mistakes can be costly.
Hybrid Agent Architectures in AI: The Best of All Worlds
Sometimes, one brain just isn’t enough.
🔧 How They Work
Hybrid agents combine multiple architectures. For example:
- A reactive layer handles immediate threats.
- A goal-based planner handles long-term strategy.
Think of it like layers of decision-making, each handling a specific aspect of the problem.
🧠 Real-World Example
- Mars Rovers: React to terrain while also following mission goals.
- Advanced Video Game AIs: React in real time, but follow strategic plans.
✅ When to Use
- Complex environments.
- When both real-time reactions and planning are needed.
⚠ Weakness
Designing hybrids can be tricky. Coordination between layers must be seamless.
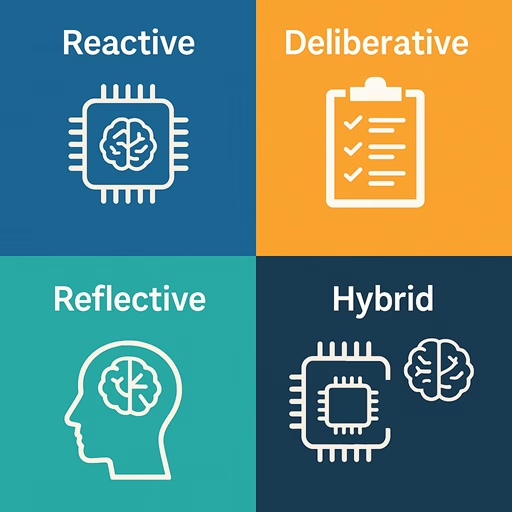
Summary Table: Agent Architectures in AI at a Glance
Architecture | Key Feature | Example | Good For |
---|---|---|---|
Reactive | Instant response | Roomba, game bots | Real-time, simple tasks |
Model-Based Reactive | Reflex + memory | Smart thermostats | Semi-smart reactions |
Goal-Based | Planning to succeed | GPS, chess bots | Strategy & navigation |
Utility-Based | Best option wins | Netflix, autonomous cars | Optimization tasks |
Learning | Improves over time | Self-driving cars, chatbots | Evolving environments |
Hybrid | Combo brains | Mars rovers, advanced AIs | Complex multi-tasking |
Final Thoughts: Choose the Brain That Fits the Job
Not every AI needs to be Einstein. Some just need to sweep your floor or recommend a snack.
But as environments grow more complex and goals more dynamic, choosing the right agent architecture becomes critical.
👉 Want something fast and cheap? Go reactive.
👉 Need strategy? Try goal-based or utility-based.
👉 Want adaptability? Bring in the learner.
👉 Need it all? Go hybrid.
Whatever your need, there’s an architecture built for it.
🔗 Related Articles
👉 AI Agents Explained: How They Think, Act, and Learn
👉 Understanding Agent Environments: Fully Observable, Stochastic & More
🌐 External Resource
Explore IBM’s AI Design Patterns for insights into real-world architectural applications in AI.